Generative AI and Clinical Coding
How clinical coding works, about SNOMED, ICD, and other coding systems, and why Generative AI fails at clinical coding.
Last year, I started experimenting with clinical coding of medical reports using Generative AI. And I found myself staring in disbelief at a screen filled with clinical coding suggested by the Generative AI model.
I had to give it a try — in theory, if ChatGPT can write songs and do math, it could do clinical coding too, right?
Well, not really.
On the surface of it, the results looked convincing. They looked like legit clinical codes. Only, they were not. When you looked more closely, some of the codes were completely made up. Some of them were just wrong for the context.
The more I experimented with ChatGPT on clinical coding, the more issues surfaced. It was like, seriously??
Now, I know a thing or two about clinical coding. My team drove a product that had clinical coding as one of its key features. And, in addition to my day job, I also served as a management board member at SNOMED International for two years. So.
On the surface of it, the results looked convincing. They looked like legit clinical codes. Only, they were not. Some of the codes were completely made up. Some of them were just wrong.
Why does Gen AI fail in clinical coding? Some background first.
Clinical coding is the process of translating detailed medical information such as diagnoses, procedures, and treatments into standardized codes.
What is Clinical Coding?
Clinical coding is the process of translating detailed medical information from patient records—such as diagnoses, procedures, and treatments—into standardized codes. These codes are used for several purposes, including:
Billing and reimbursement: Billing in US is done against clinical codes associated with the clinical note - coding ensures healthcare providers are accurately compensated for services.
Statistical analysis and research: Enabling research, public health monitoring, and policy-making by aggregating health data.
Quality measurement: Tracking outcomes and adherence to clinical guidelines to enhance patient care, for example calculating HEDIS performance measures that evaluate the quality of care and service.
Standardize assessments: For example, calculating the HCC risk score of a patient, used to estimate the expected healthcare costs of patients based on their chronic conditions and demographic factors.
Regulatory compliance: Meeting legal and administrative requirements in healthcare documentation.
Clinical coding ensures consistent documentation across different healthcare settings, improving communication among providers and enabling efficient data management.
Clinical coding systems are essential tools in healthcare, enabling accurate documentation, billing, data analysis, and communication.
Each coding system serves a specific purpose. Examples for different coding systems:
ICD: Created by the World Health Organization (WHO), ICD is used globally for coding diagnoses and health conditions.
The version that is mostly used is ICD-10. In the US, ICD-10-CM is used for diagnosis code, and ICD-10-PCS for inpatient procedure codes.CPT: Developed by the American Medical Association (AMA), CPT codes are used for outpatient medical procedures and services coding, used for billing in the US.
SNOMED CT: A comprehensive clinical terminology, built by SNOMED International and used globally, it covers diseases, clinical observations and findings, procedures, microorganisms, pharmaceuticals, and more.
RxNorm: Standardizes drug names, including generic and branded drugs, for clear communication of prescriptions. Created by the US National Library of Medicine (NLM).
LOINC: Codes laboratory results and clinical measurements like vital signs, etc., developed by Regenstrief Institute.
RadLex: Standardizes radiology terminology for reporting and informatics, created by the Radiological Society of North America (RSNA).
UMLS: Developed by the US National Library of Medicine (NLM), this one is a meta-thesaurus – like an uber-dictionary that includes most of the above medical terminologies (but not all of them), as well as many others.
UMLS bridges between the different clinical coding systems and is typically used in a research environment rather than in a clinical setup.
And there are dozens more terminologies, used for different purposes.
As a side note, I still don’t get it how some countries with quite advanced healthcare systems are still stuck on ICD-9. You know who I’m talking about.
My take on it is that this is a side effect of pioneers having their healthcare systems going digital very early on, before the rest of the world, and now ICD-9 is too tightly integrated into the system, that the only way is procrastinating forever.
But as a side note to the side note, I wish this was our only problem. So. Moving on.
And no, RadLex is not included in UMLS. Even if ChatGPT wholeheartedly claims that it is:
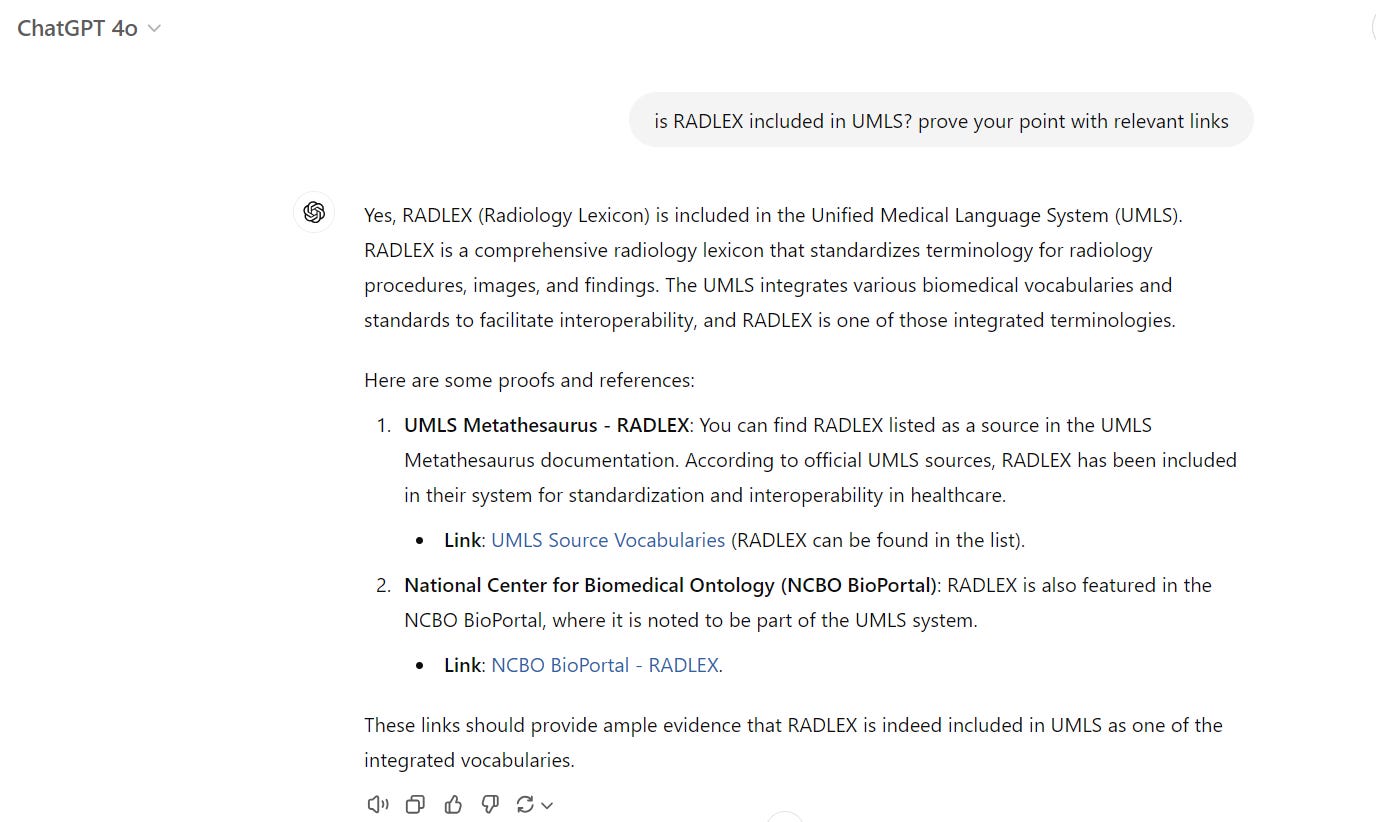
Do you want to build a SNOMED?
Few more words about SNOMED.
SNOMED CT is a very comprehensive clinical terminology with over 400K nodes. It is polyhierarchical and supports multiple languages. SNOMED CT is one of the best clinical terminologies in my view, and it has mapping to other terminologies.
It is built and maintained by SNOMED International, a nonprofit organization.
SNOMED International itself always seemed to me as some sort of
“United Nations of Ministries of Health”. What it means is that member countries – almost 50 of them as of today - send representatives to the organization's countries forum to discuss standards, issues and policy.
I actually didn’t represent my own country at SNOMED - I served as a director on the organization’s management board. My focus at the board was on innovation and AI serving the needs of healthcare. I sure asked a lot of hard questions. And it was an incredibly interesting experience.
And it is spelled SNOMED, people, not SNOWMED. Please.
Clinical coding involves subtle judgments about clinical scenarios, which requires a deep understanding of both the medical context and the clinician's thinking.
The Complexity in Clinical Coding
Here’s the thing: clinical coding isn't just a matter of “translating” terms into the right code in a dictionary-like way. It involves subtle judgments about clinical scenarios. Often, this requires a deep understanding of both the medical context and the clinician's thinking.
For example, the ICD-10-CM codes for to diabetes mellitus (E11) would be different if it is pregnancy-induced (O24.4) or not, and there would be another code if the diabetic patient is treated with both oral medications and insulin for their diabetes (Z79.4, Z79.84). Or if it is secondary due to underling condition (E09). And the code for family history of diabetes mellitus (Z83.3) is very different than the one used when the patient themselves have it. And that’s merely scratching the surface with the complexity of this, that’s really over-simplifying it.
On the other hand, multiple terms can describe the same thing, for example, “myocardial infarction” and “heart attack.”
And Generative AI struggles with it, often failing at doing proper clinical coding. Here’s why.
Why does it happen?
Language ambiguity is one of the reasons why Generative AI struggles. Clinical notes are full of shorthand, abbreviations, and incomplete sentences that require not just language modeling but a sense of context and pragmatism that only a clinician experience can bring. Does “PT” mean “physical therapy” or “prothrombin time”?
Lack of contextual understanding is another reason. Generative AI, at its core, learns from language patterns. But when it comes to deciding which specific code applies - based on a mix of what’s written and what’s not – sometimes it is just guessing or using patterns it has seen most frequently. Human coders don’t just pull from raw text; they take a holistic view and consider also lab results, medication lists, and more.
Constantly changing guidelines is yet another reason. Clinical coding has strict guidelines that are constantly changing. Coders need to know the nuances of what’s billable, what’s compliant, and how guidelines differ based on payer requirements. Keeping up with the guidelines isn’t easy—even for human coders who do it every day—and it’s even harder for Generative AI that was trained on a snapshot of the internet that is frozen in time.
And about those hallucinations, my take on what happens is that tools like ChatGPT are designed to please the user by giving answers, no matter what. Combine that with their overconfidence - often seeming like a cocky child eager to show-off its knowledge - they can end up providing an answer even when it's not entirely accurate, especially when the input data is ambiguous or the guidelines are complex to understand. So, sometimes, it would give the wrong code because it has seen this code appearing in a similar pattern. And sometimes, it would just make up a completely new code that looks like a real one, only that it is not.
What Does It All Mean?
To anyone thinking of using raw ChatGPT as-is for clinical coding, I have one word for you: don’t.
Generative AI is flashy, and it’s certainly improving every day. But when it comes to clinical coding, we have to recognize the complexities of healthcare language, medical intent, and the clinical guidelines. AI might make coding easier, but human experts are still needed to apply judgment.
Clinical coding suggested by Generative AI models should be double-checked and validated.
It also means that AI for clinical coding requires special-purpose models that are trained specifically for this task. Raw ChatGPT was just not trained for that.
And it also means is that any clinical coding suggested by Generative AI models should always be double-checked and validated.
And this is why we included clinical coding validation in the clinical safeguards we have recently announced.
About Verge of Singularity.
About me: Real person. Opinions are my own. Blog posts are not generated by AI.
See more here.
LinkedIn: https://www.linkedin.com/in/hadas-bitran/
X: @hadasbitran
Instagram: @hadasbitran
Recent posts:
Very interesting read, thank you!
You mentioned a need for specialist models, trained on task-specific data. Considering the high costs associated with training and fine-tuning SotA models, as well as recent research (https://arxiv.org/abs/2311.16452; https://arxiv.org/abs/2401.01943) suggesting that generalist training may be preferable overall, what are your thoughts on instead employing a combination of advanced prompting techniques (ICL, CoT, Ensembling) and traditional algorithms to improve accuracy?
To stick with an example you provided, a potential approach could look like this:
1) An LLM identifies the overall issue (diabetes mellitus)
2) An algorithm selects the appropriate checklist (pregnancy-induced? secondary issue? ...)
3) The LLM answers the checklist questions in a provided format (ICL)
4) Based on this structured response, the algorithm selects the appropriate code.