Can Generative AI disrupt Clinical Trials?
How a White House Presidential Innovation Fellows challenge led to a unique adventure, what happened at the Bill G review, and how Generative AI can improve patient access to clinical trials.
This is the unusual story of Project New Hope.
Our team started an AI project for Clinical Trials Matching back in 2017 as part of a hackathon. We named the project A New Hope, after the film.
Side note for those of you who are not in the tech industry: the Star Wars universe is a thing. It has always been a source of inspiration and cultural references for the tech industry. This is The Way.
A New Hope
A New Hope was a Natural Language Processing-based AI system that was designed to understand the eligibility criteria of clinical trials and check if a patient could be potentially suitable.
Few words on Natural Language Processing (NLP). It is an area in AI that helps systems understand and interact with the human language. It’s like teaching the machine to understand the way humans naturally speak, write and generate human language. It is a broad term and includes things like understanding the intent of the speaker, recognizing part of speech in a sentence, understanding sentiment, disambiguating meaning of words, extracting keywords, and even creating summaries, generating responses, and beyond.
A New Hope aimed to understand the language of the protocol that describes a clinical trial, analyze the inclusion and exclusion criteria, and cross-reference that against the patient’s medical information, labs, etc. to check if there’s a potential match, and if a piece of information was missing, ask for it.
Matching patients to clinical trials is a big problem. Clinical trial protocols are complex and typically have dozens of complicated inclusion and exclusion criteria, expressed in medical language, designed to support of the trial success. There are tens-of-thousands of clinical trials around the world that are recruiting patients at any point in time, more are added every year, but most trials are unable to reach the target number of recruited patients, which causes delay in bringing new drugs and therapies to the market.
For patients, finding a clinical trial that would be the right match could give them access to a new drug or treatment. For some patients, this could mean a new hope.
The Bill G Review
It was early 2018 when I presented this technology in a review with Bill G. He had a mixed reaction to it. His response was – but it is a hard problem, acknowledging the importance of finding a solution, but doubting that the technology would ever be able to get it right. Bill G is sharp, his reviews go deep, and he asks hard questions. It is often a humbling experience, you really need to know what you’re talking about.
However, that review was not the most daunting presentation in this story. The review ended safely, which wasn’t the case for some of the other reviews that day.
The White House Presidential Innovation Fellows Challenge
In October of the same year, the White House Presidential Innovation Fellows launched a challenge around AI in Health Tech, aiming at improving clinical trials recruitment. We decided to take part in the challenge and evolve the clinical trials matching technology. As part of that, we received a de-identified dataset from the National Cancer Institute (NCI), representing data of patients that were a potential match against specific trials.
The dataset included structured eligibility criteria, data of patients that were considered for those trials, and some Ground Truth: patient-to-trial match that was curated by medical professionals – is there a match or not, and if not, which criteria failed.
Few words on Ground Truth: in Health Tech, it is common to have clinical staff and medical professionals as part of the R&D team, creating such Ground Truth datasets that are adapted to the task you want to have the AI system accomplish. That Ground Truth represents what the correct answer should be from the clinical perspective, and this is how you measure the performance of your AI system.
Using the datasets from NCI, our team then created a solution that included a conversational AI experience powered by an algorithm that analyzes the trials criteria and matches those against the structured de-identified patient data.
The unbelievable yet true story of Washington DC
We were one of the winning teams in that challenge. At the end of February 2019, we were invited to take part in a US Census Bureau event in Washington DC, and present our work at the White House.
And so, after submitting all the required paperwork for security clearance to get into the White House, I was heading to Washington DC, together with two of my data scientists, Danny Karmon and Shahar Admati.
It was the night before the presentation. The three of us were already in Washington DC. I just finished giving an interview to Bloomberg, when I received a call from one of the Presidential Innovation Fellows we were working with, telling me that our security clearance did not come in yet, likely due to some random delay on our home country’s side, and that we should try to find someone else from our company that has a US citizenship, to represent our work at the White House the next day. Devastating.
There was no time to mourn over this, we had to get our act together. It was already after 9pm and we were supposed to enter the White House for the presentation the next morning at 7am. We moved to execution mode, splitting the mission between us. Danny was calling our country’s Ministry of Foreign Affairs situation room, telling them that 3 scientists needed an immediate security clearance for a presentation at the White House the next morning. I went for the more practical plan, desperately looking for a local backup that could come in and represent the company instead of us at such a short notice. The only local person I could get a hold of was Shannon, from our field operations in DC. She had no background or context on the project, but she could make it.
Shannon arrived at our hotel at 1am. We sat together until 3am that night, with me walking Shannon over the project and going into the technical and scientific details. She took a copy of the presentation and we agreed to meet the next morning at 7am at the back entrance of the White House.
We did not hear back from the situation room of the Ministry of Foreign Affairs of our country, but decided to try going anyway, just in case a miracle has happened overnight.
And as unbelievable as this may sound, that’s exactly what happened. Our security clearance arrived during that night, and we got into the White House. Sweet like justice, karma is a queen.
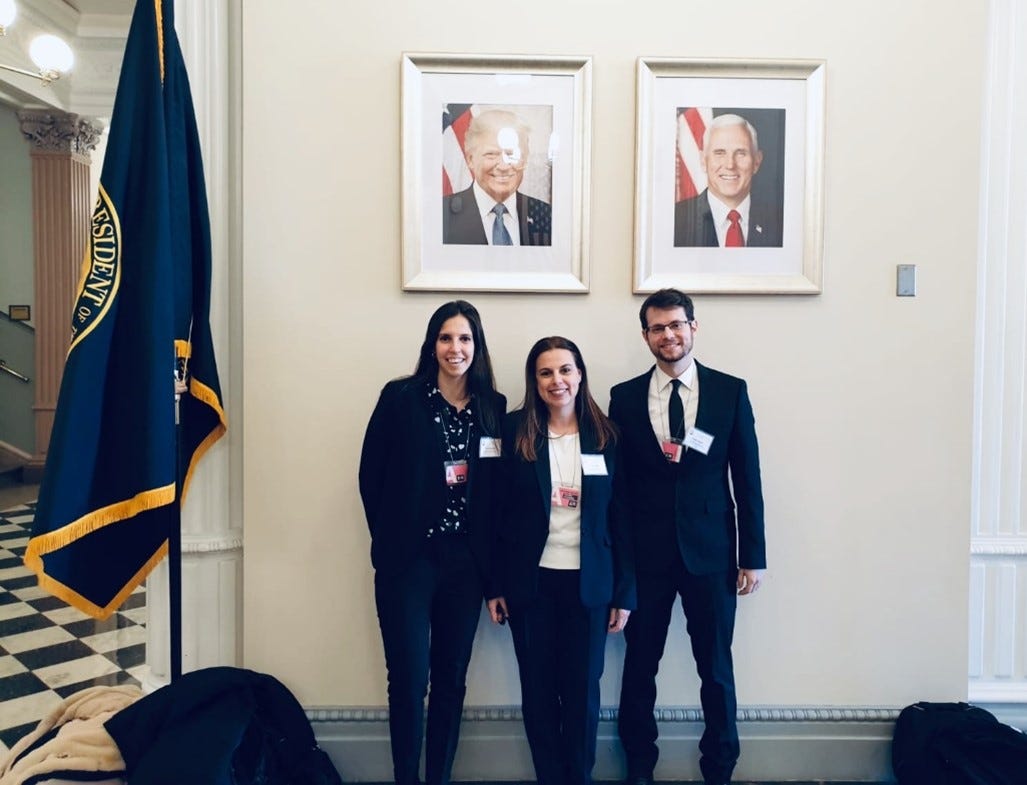
The presentation took place at the Indian Treaty Room, located in the East Wing of the White House, in the presence of HHS CTO and Chief Information Officer, FDA representatives and other senior leaders of the US healthcare system. Less than a hundred attendees. I often engage in presentations to larger audiences, sometimes even do keynotes at conferences - and still, this one was, by far, the most daunting presentation I ever gave.
Generative AI in the service of Clinical Trials
The project was renamed to Project New Hope, to follow company naming standards that use city names. Project New Hope finally went live with several customers in 2020. It continued to evolve, and in the recent years has become one of the decision support models in a service called Azure Health Insights.
One of the more intriguing directions of evolution is the potential use of Generative AI to further improve the process of matching patients to clinical trials. Assisting the trial matching process of inclusion and exclusion criteria against patient information from their medical records, has traditionally been a time-consuming and costly process, and can potentially be further improved. Interacting with patients over a chat experience to ask questions related to eligibility criteria can become better and more engaging with Generative AI. Simplifying the information about the clinical trial entry criteria and process can make the trial more accessible for potential participants. Those are just a few examples for technologies that are already available today and can be further improved with Generative AI.
And there is more. Generative AI can optimize the process for authoring protocols for clinical trials, and help find relevant data in scientific literature. Generative AI can also help summarize large amounts of data that are collected during the trial to streamline the operations involved in conducting clinical trials. With the evolution of Generative AI toward models using multiple modalities, there will likely be additional ways technology can contribute to more efficient trials with greater success rates.
It is important to note though, that while Generative AI can greatly contribute to patients access to clinical trials, it also has its limitations and challenges. Healthcare is a sensitive domain, and can’t afford inaccuracies or relying on insufficient evidence. Generative AI also needs to be presented with guardrails for not fabricating things that do not appear in the original data, a phenomena we call hallucinations. It becomes critical to build defense mechanisms to cope with those issues. We continue to apply Responsible AI and deployment safety processes into everything we do.
The direct conclusion here is that human oversight of the clinical trials matching process is crucial. Results would always need to be validated by a human clinician before taking decisions such as accepting a candidate to a clinical trial.
Read previous posts:
About me: Real person. Opinions are my own. See more about me here.
About Verge of Singularity.
LinkedIn: https://www.linkedin.com/in/hadas-bitran/
X: @hadasbitran
Instagram: @hadasbitran